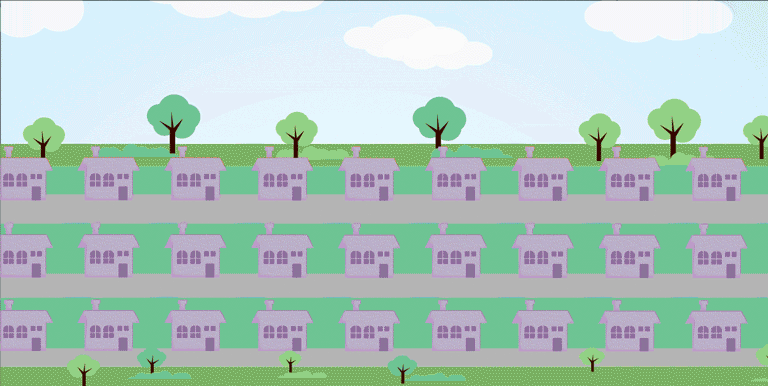
Home in on your Ideal Audiences
One of the things brand marketers tell me they like about third-party data is the way it allows them to mix and match attributes so they can home in on their ideal audience.
Want to reach high-earning men who’ll buy a high-end sports car model? No problem, buy an auto-intender’s segment, overlay it with a gender segment, and to ensure you target men who can afford the sports car, purchase a creditworthiness segment from a company like Experian.
Sounds good, except for a few things. Let’s start with the auto-intender segment. Can you trust it? How was the data collected, classified, and scored? How many data points were used to determine that a consumer is truly in-market for a car, and not simply engaging a life-long habit of reading about new cars? Did the data vendor collect that data itself, or purchase it from somewhere else? How recent is that data, and how do you know if those consumers haven’t already converted? Who else has that segment been sold to? Will you be competing with multiple brands for the same pool of users?
Now apply those same questions to the additional datasets you plan to overlay — gender, creditworthiness, and so on.
0 to 50% accuracy is just the start of your problems
How accurate is that data anyway? One way to find out is to ask consumers whether or not the data companies got it right, which is pretty much what Deloitte did. According to a Deloitte survey of consumers, 59% of respondents said that the accuracy of the demographic data collected on them was somewhere between 0 – 50% correct. Another 44% said the information about their vehicles was 0% correct, while 75% said the vehicle data was between 0 – 50% correct.
Not a good track record.
Then there’s the whole question of legality … did those data providers get the consumer’s consent to collect and sell their data? Are you confident that consumers haven’t opted out somewhere along the supply chain? Is their data receiving the full protection afforded them under GDPR and CCPA?
These are thorny issues, brand marketers tell me. But can household characteristics mix and match attributes with the same precision as third-party data? The answer is yes, only it’s far less “theoretical” than third-party data. And it won’t land you in hot water with the regulators either.
Digiseg audience overlays and composite audiences
There are all sorts of characteristics that determine what a consumer will need — lifecycle, income level, neighborhood type, education level, tech-savviness, to name just a few.
Digiseg’s data modeling combines dozens of data points — all from trusted sources — to help you find consumers who will be interested in your brand because they actually have a need for your products and services. They might not make a purchase immediately, but they will eventually.
Over the past year alone, brand marketers have used our audience segments to home in on people who are:
- Budget conscious but still keen to try the next best mobile service
- Dog parents
- Appreciative of clean cars but have little inclination to do the work of scrubbing and vacuuming themselves
- Willing to pre-order an electric car sight-unseen
I’m actually not kidding.
How’d we do it? No household characteristic is an island. Wealthy households with a high level of education tend to have higher than average tech levels. Households in urban areas tend not to own multiple cars. By pairing multiple characteristics, we too can home in on an ideal audience.
The big difference is that our audience segments are far more accurate, as they’re composed of data that are gathered, vetted, maintained, and anonymized by National Statistics Offices across Europe and the U.S. And our data doesn’t use cookies, so our segments are inherently privacy compliant.
Finally, we helped an automaker identify and reach consumers most likely to pre-order an electric car sight unseen (try doing that with third-party data!).
We created this custom audience by selecting consumers who already have a vehicle — a clear indication that they need and use a car on a regular basis. Given the limited range of most electric cars at present, we also focused on consumers who live in suburbs and cities, where the distances they drive are shorter and charging stations are more plentiful. Finally, we focused on the top 40% of the wealthiest households as they are the consumers most likely to preorder a vehicle without test driving it first.
All four campaigns exceeded the goals laid out by the brands, and vastly outperformed the traditional third-party data sets the brand marketers also used.Why do audience segments built on household characteristics perform better?
Household characteristics begin with a validated need. Do these consumers live in an area where cars are necessary for survival? Do they live in an area where parking is widely available (meaning they have houses that can easily accommodate multiple automobiles as opposed to apartment buildings with limited parking).
These needs tend to be very stable; people who live in a neighborhood that’s predominantly made up of single-family homes are likely to stay there until the kids are grown and they’re ready to downsize.
By mixing and matching these characteristics, we can help brand marketers reach their ideal audience at scale. In fact, our growing list of composite audiences (which are based on seeing what works and what doesn’t through the years) make quick of targeting niche audiences, such as city dwellers who prefer micro-cars or consumers looking for a loan to finance a household renovation.
And that’s how we bring the message “home” for brand marketers.
/ Søren H. Dinesen, CEO Digiseg – Shd@digiseg.io